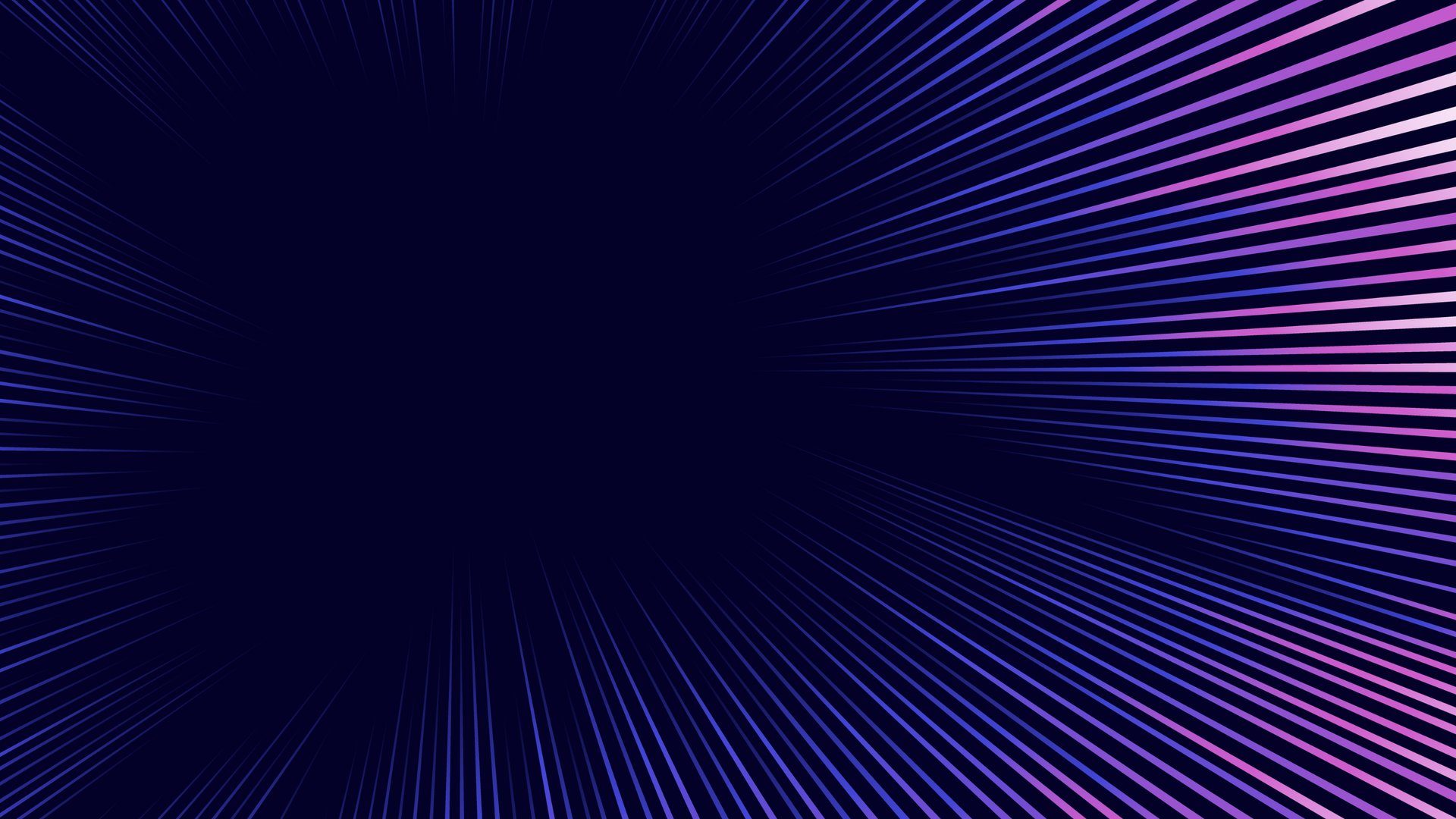
Master Predictive Analytics: Your Guide to Data-Driven Decisions
Learn the basics of predictive analytics in our essential guide to data-driven decision making. Understand how to leverage data to make informed decisions and improve business outcomes. Master the fundamentals of predictive analytics with our comprehensive overview.
Waqar Jadoon
2/25/20247 min read
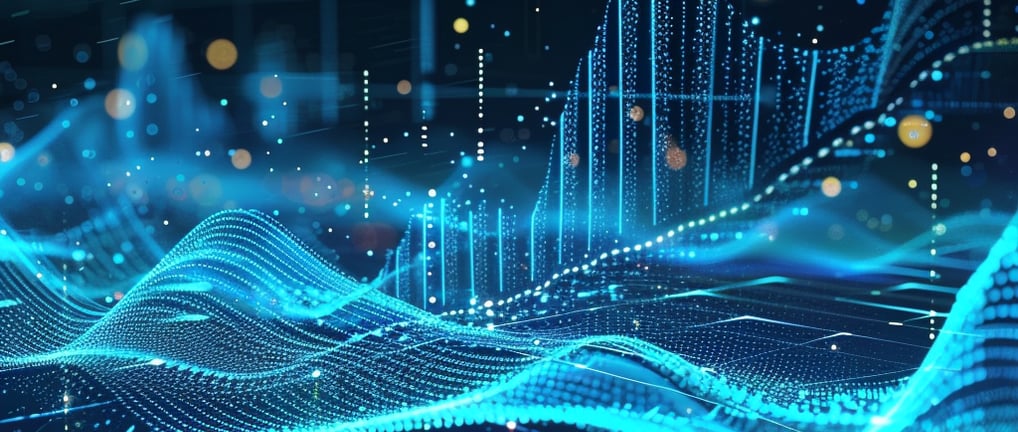
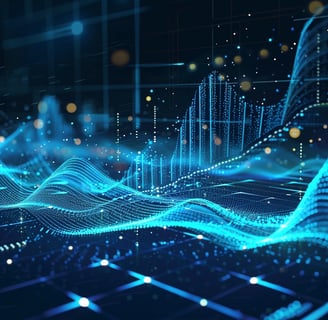
Introduction to Predictive Analytics
Predictive analytics is a strategic tool that leverages data, statistical algorithms, and machine learning techniques to forecast future outcomes based on historical data patterns. By analyzing past trends and behaviors, organizations can gain valuable insights to make informed decisions, mitigate risks, and optimize opportunities. In today's data-driven landscape, predictive analytics plays a pivotal role in enhancing business intelligence and driving competitive advantages.
The essence of predictive analytics lies in its ability to transform raw data into actionable insights, enabling organizations to proactively respond to changing market dynamics and customer preferences. By identifying trends, patterns, and correlations within datasets, businesses can anticipate future trends, make accurate forecasts, and tailor their strategies for optimal results. Embracing predictive analytics empowers companies to make evidence-based decisions, streamline operations, and stay ahead of the curve in an increasingly competitive business environment.
Fundamentals of Predictive Analytics
Predictive analytics is founded on the principles of leveraging historical data to forecast future outcomes. At its core, this discipline involves the application of statistical algorithms and machine learning techniques to identify patterns within the data that can be used to make informed predictions. By understanding the fundamental concepts of predictive analytics, organizations can harness the power of data to make strategic decisions and enhance operational efficiency.
Machine learning plays a pivotal role in predictive analytics by enabling algorithms to learn from data, identify trends, and generate predictive models. Through the process of training these models on historical data, organizations can gain valuable insights into potential future scenarios. Understanding the fundamentals of machine learning is essential for building accurate predictive models that can assist in everything from financial forecasting to customer segmentation.
Data Collection and Preparation
Data collection is a critical step in the realm of predictive analytics, where the quality and quantity of data can significantly impact the accuracy of predictive models. In this phase, companies leverage a variety of tools and technologies to gather relevant data from both internal and external sources. Advanced techniques in Artificial Intelligence (AI) are instrumental in automating data collection processes and ensuring the acquisition of diverse datasets for analysis.
Once the data is collected, the next phase involves cleaning and preparing the information for predictive modeling. This stage includes tasks such as handling missing values, removing outliers, and standardizing data formats to enhance the consistency and accuracy of the dataset. AI algorithms play a crucial role in data preparation by streamlining these processes and detecting patterns within the data that can be used to enrich predictive models further. By adhering to best practices in data collection and preparation, organizations can lay a strong foundation for deriving valuable insights through predictive analytics.
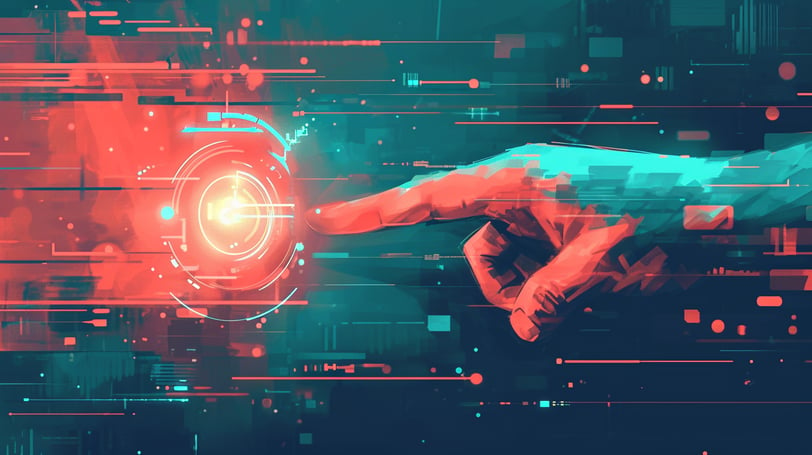
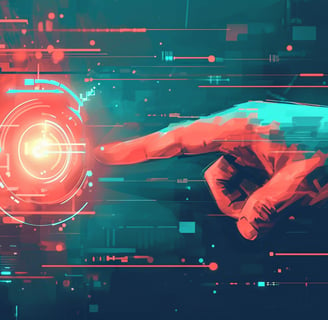
Common Predictive Analytics Techniques
Predictive analytics techniques are essential tools in the realm of data analytics, aiding in the extraction of valuable insights and trends from vast datasets. One common technique is regression analysis, which involves analyzing the relationship between dependent and independent variables to predict outcomes. By utilizing regression models, businesses can forecast sales, understand customer behavior, and optimize marketing strategies based on historical data patterns. This technique is particularly useful in scenarios where understanding the impact of various factors on a target variable is crucial for decision-making.
Another prevalent method in data analytics is classification, which involves categorizing data points into predefined classes based on their attributes. This technique is widely used in sentiment analysis, fraud detection, and customer segmentation. By employing classification algorithms like decision trees, support vector machines, or logistic regression, businesses can enhance their decision-making processes by accurately classifying data points and predicting future outcomes based on historical patterns. Mastering classification techniques empowers organizations to leverage data effectively and make informed strategic choices in a competitive landscape.
Building Predictive Models
When constructing predictive models, it is essential to start with a thorough understanding of the data at hand. Proper data preparation and cleansing are crucial steps in ensuring the accuracy and reliability of the models. By conducting exploratory data analysis and utilizing data visualization techniques, such as scatter plots or histograms, analysts can gain insights into the distribution and relationships within the dataset. This initial stage sets the foundation for building robust predictive models that can effectively leverage the patterns and trends present in the data.
Once the data has been adequately prepared, the next step in building predictive models involves selecting the appropriate algorithms and techniques based on the nature of the problem and the desired outcomes. Various techniques, such as regression analysis, decision trees, and neural networks, can be employed to develop predictive models that can effectively forecast future trends or make informed decisions. It is crucial to evaluate the performance of the models through metrics like accuracy, precision, and recall to ensure they are meeting the desired objectives. By iteratively refining the models based on feedback and validation, analysts can create predictive models that are reliable and actionable.
Interpretation and Visualization of Predictive Results
Interpretation and visualization of predictive results are crucial steps in the predictive analytics process. Once predictive models have been built and applied to the data, it is essential to carefully analyze the outcomes to draw meaningful conclusions. The interpretation of results involves understanding the relationships between variables and how they influence the predicted outcomes. This phase requires a keen eye for detail and an analytical mindset to uncover insights that can drive informed decision-making.
Moreover, visualization plays a key role in communicating the findings of predictive analytics effectively. By presenting data visually through graphs, charts, and other visual aids, complex insights can be simplified and understood more easily by stakeholders. Visual representations of predictive results can highlight patterns, trends, and anomalies that might not be as apparent in raw data, enabling decision-makers to grasp the implications of the analysis at a glance. In this way, interpretation and visualization work hand in hand to extract actionable insights from predictive models and enhance their practical utility in various applications.
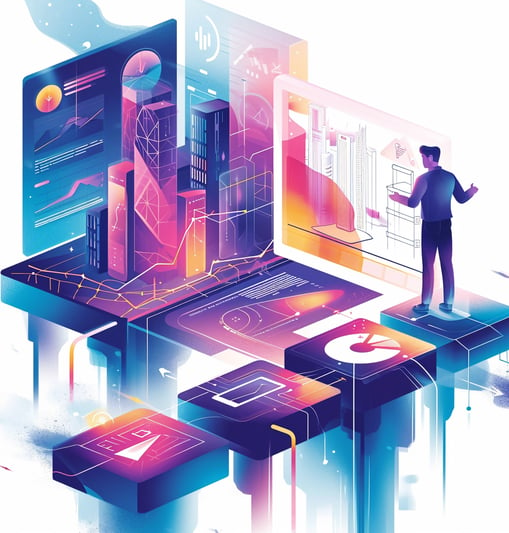
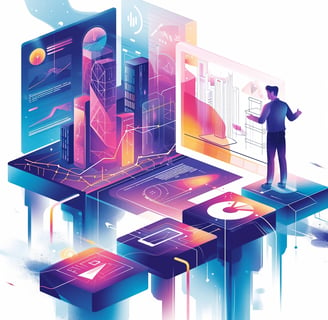
Applications of Predictive Analytics
Predictive Analytics is widely utilized in various industries such as finance, healthcare, marketing, and retail, revolutionizing decision-making processes by predicting future outcomes based on historical data. In finance, predictive analytics plays a crucial role in forecasting stock prices, detecting fraudulent activities, and managing risks. By analyzing vast amounts of data, financial institutions can optimize investment strategies and enhance customer experience through personalized services.
Healthcare professionals leverage predictive analytics to improve patient outcomes, optimize treatment plans, and reduce hospital readmission rates. By analyzing patient data, healthcare providers can identify high-risk individuals and intervene proactively to prevent health complications. Moreover, predictive analytics assists in streamlining operational processes, ensuring efficient resource allocation, and improving overall healthcare delivery.
Challenges and Considerations
Meeting the evolving demands of predictive analytics poses a considerable challenge for organizations today. With the exponential growth of data volumes, ensuring data quality and consistency becomes paramount. The need for skilled professionals proficient in statistical modeling, data analysis, and machine learning is a critical consideration in deploying successful predictive analytics initiatives.
Furthermore, the presence of biases in data can skew predictive outcomes, leading to inaccurate insights and flawed decision-making. It is imperative for organizations to implement robust strategies for identifying and mitigating biases in data collection and analysis processes. Ensuring transparency in predictive models and adhering to ethical standards in data usage are foundational elements in overcoming these challenges within the realm of predictive analytics.
Tools and Resources for Predictive Analytics
For professionals engaging in predictive analytics, having access to the right tools and resources is crucial for successful outcomes. Utilizing software such as Python, R, or SAS can aid in data processing, model building, and result interpretation. These platforms offer a wide range of functionalities, including statistical analysis, machine learning algorithms, and visualization tools, enabling users to effectively analyze large datasets and generate accurate predictions.
In addition to software, professionals can benefit from online resources and communities dedicated to predictive analytics. Platforms like Kaggle, GitHub, and Stack Overflow provide a wealth of information, code repositories, and forums where experts share their knowledge and experiences. Engaging with these communities can help users stay updated on the latest trends, troubleshoot challenges, and collaborate with peers to enhance their predictive analytics skills.
Future Trends in Predictive Analytics
As the field of predictive analytics continues to evolve, advancements in machine learning algorithms are expected to play a pivotal role in shaping its future. Techniques such as neural networks, deep learning, and natural language processing are anticipated to become more prevalent in predictive modeling processes. These sophisticated algorithms will enable professionals to extract valuable insights from vast amounts of data, ultimately enhancing the accuracy and efficiency of predictive analytics solutions.
Moreover, the integration of artificial intelligence (AI) into predictive analytics tools is set to revolutionize the way businesses leverage data for decision-making. AI-powered systems will offer real-time predictive capabilities, allowing organizations to proactively address potential risks and capitalize on emerging opportunities. By harnessing the power of AI-driven predictive analytics, professionals can gain a competitive edge in today's data-driven landscape.
What is predictive analytics?
Predictive analytics is the practice of extracting information from existing data sets in order to determine patterns and predict future outcomes and trends.
What are some common predictive analytics techniques?
Common techniques include regression analysis, decision trees, neural networks, clustering, and time series analysis.
How important is data collection and preparation in predictive analytics?
Data collection and preparation are crucial in predictive analytics as the accuracy and reliability of the predictions heavily rely on the quality of the data used.
What are some applications of predictive analytics?
Predictive analytics is used in various industries such as finance, marketing, healthcare, and manufacturing for tasks such as risk assessment, customer segmentation, fraud detection, and demand forecasting.
What are some future trends in predictive analytics?
Some future trends in predictive analytics include the use of artificial intelligence and machine learning algorithms, increased focus on real-time analytics, and the integration of predictive analytics into business processes for more actionable insights.
About the Author:
Waqar Jadoon, with 28 years in aviation supply chain management, holds an MBA and a software engineering degree. Renowned for streamlining operations and integrating AI and data analytics, he blends technical and business expertise to drive industry innovation. Waqar champions sustainable practices and technological advancements, shaping the future of aviation logistics.
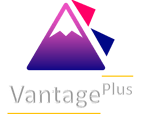

Location
Business Center 1, M Floor. The Meydan Hotel, Nad Al Sheba, Dubai U.A.E
Hours
Monday - Sunday 08:00am - 08:00pm
Contacts
Phone: +971566121732 info@vantageplusanalytics.com
waqarjadoon@vantageplusanalytics.com
FAQ