Explainable AI (XAI) for predictive models: Building trust and transparency by explaining the reasoning behind predictions.
Explore our in-depth blog post on Explainable AI (XAI) for predictive models, a critical tool that fosters trust and transparency by elucidating the logic behind AI predictions. Enhance your understanding of how XAI is revolutionizing decision-making processes across various industries.
Waqar Jadoon
4/4/20249 min read
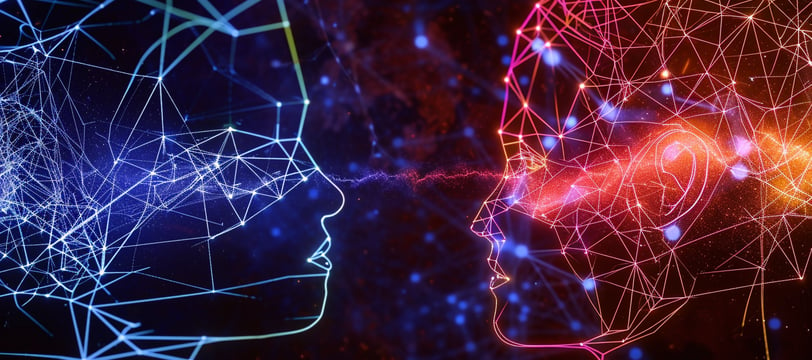
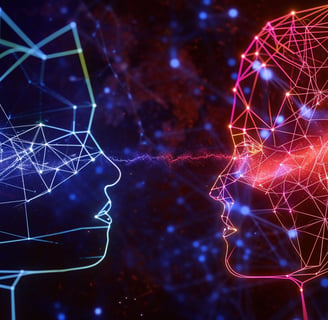
Understanding the Concept of Explainable AI
Explainable AI (XAI) is a subfield in artificial intelligence that focuses on constructing AI models that human stakeholders can comprehend. Given the complex and often inscrutable nature of traditional neural networks and machine learning models, the need for XAI has never been more evident. This branch of artificial intelligence is crucial for making AI-driven systems more accountable, trustworthy, and effective by providing insights into why particular results are generated.
The primary aim of XAI is to make artificial intelligence's decision-making process more clear and understandable to humans. This involves designing machine learning models that can not only perform sophisticated tasks but also articulate their operations and predictions in a manner that humans can understand. By doing so, XAI facilitates a deeper, holistic comprehension of complex decision-making processes. This allows users to harness the potent predictive power of AI while ensuring that these systems are accountable and transparent.
The Role of Transparency in Predictive Models
Transparency is a fundamental criterion for evaluating the effectiveness and reliability of predictive models. An ideal predictive model not only delivers accurate predictions, but also provides insight into the statistical correlations and computational algorithms that enable its forecasts. This fusion of efficiency with interpretability is known as Predictive Models Transparency, a critical component that determines the model's ability to establish trust among its users.
Predictive Models Transparency advances the understanding of how machine learning algorithms function, thus reducing the black-box nature of these systems and promoting verifiability. It offers users a glimpse into the engine room, illuminating how input variables have been manipulated and weighted. Transparency in predictive models allows for more objective verification, fosters trust, and ensures the system's decisions are ethically and legally sound. The result is a system that not just predicts, but also explains, ensuring a robust synergy between human decision-making and artificial intelligence.
Establishing Trust in Artificial Intelligence Systems
As the proliferation of Artificial Intelligence (AI) amplifies in various sectors ranging from healthcare, finance, marketing to everyday consumer technology, building trust in AI becomes a linchpin for its successive adoption. It is paramount that these AI systems meet the benchmarks of reliability, fairness, and comprehensibility to ensure their acceptance. AI-driven tools should be articulate in their functioning, offering the ability to comprehend and reproduce the decision-making journey. This would aid in dispelling any aura of ambiguity linked with AI, fostering an environment of trust and confidence among its users.
In this quest of building trust in AI, the dual factors of transparency and interpretability play a pivotal role. While transparency refers to the disclosure of all influential variables and steps involved in a prediction, interpretability is the extent to which a human can understand the cause of a decision taken by the AI model. Emphasizing on these characteristics not only fortifies the trust quotient but also encourages the accountability of AI systems. Moreover, organizations can benefit by enhancing their customer relationship through this undeniable trust, thereby earning loyalty and favorability for their AI-driven solutions.
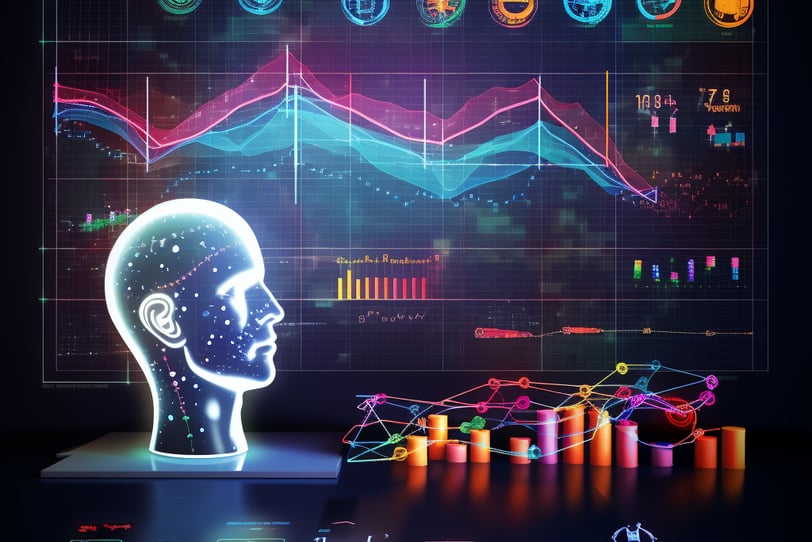
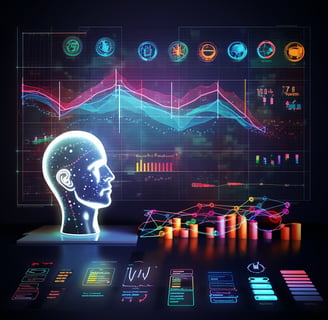
Decoding the Decision-Making Process in AI
Artificial intelligence systems are continually evolving, making complex decisions based on intricate algorithms and vast amounts of data. An essential component of these systems is the ability to provide understandable explanations of this decision-making process, also known as AI Decision Explanation. This concept delves into the rationale behind each decision made by an AI system, illuminating its pathway from input to output. It aids in understanding how and why a particular decision was reached, making the entire process less of a 'black box' and more transparent.
On the other hand, AI Decision Explanation serves a critical role in not just understanding but also refining these automated decision systems. By decoding these decisions, developers can identify and rectify any bias, errors, or inconsistencies in the system's predictions, thereby improving its overall performance. Through this process, AI becomes a tool that can make finely tuned decisions with utmost accuracy while maintaining full transparency.
Key Techniques in Implementing Explainable AI
The realm of artificial intelligence has seen a significant shift with the introduction of XAI Techniques. These techniques are instrumental in making the operations of AI systems much more transparent and logical to human understanding. They are applied in various areas such as natural language processing, image recognition, and predictive analytics, providing comprehensive insights into the results drawn by AI algorithms.
XAI Techniques involve several methods that help to break down and interpret the decision-making processes of AI systems. Primarily, these include feature attribution, surrogate models, and counterfactual explanations. Feature attribution focuses on identifying the influence of each feature in the prediction or decision made by an AI system. Surrogate models attempt to create an interpretable model that behaves similarly to the original black-box model, revealing its decision-making process. On the other hand, counterfactual explanations provide an alternate scenario explaining how different results could be achieved by altering certain inputs. By leveraging these techniques, we can ensure the implementation of an effective and transparent AI system.
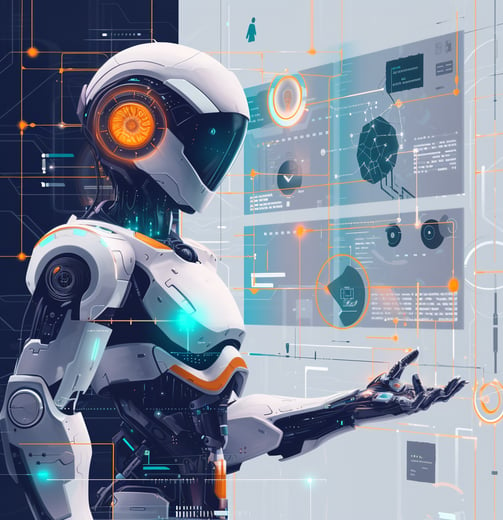
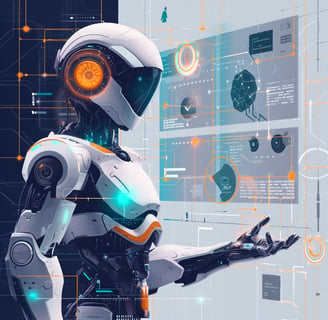
Importance of Transparency in Machine Learning
The profound influence of transparency in machine learning cannot be underestimated as the field charges ahead to dominate various industries. By rendering machine learning models more transparent, we provide a clear window into how these powerful tools generate results, which proves critical in certain operational contexts. Transparency ensures that stakeholders at all levels understand the basis for AI decisions, fostering a higher degree of accountability and fostering trust in these advanced systems. This paves a path for better acceptance and broad-scale adoption of AI-enabled processes across sectors.Importance of Transparency in Machine Learning
Simultaneously, transparency in machine learning enhances the robustness of AI applications by facilitating better error detection and system debugging. With explainable mechanisms, programmers can easily trace back steps to identify and rectify any potential issues that may influence the model's behavior, thereby refining its predictive accuracy. This, in turn, leads to more reliable decision-making processes, especially for use in critical areas such as healthcare, finance, and public policy, where the ramifications of inaccurate predictions can be significant. Hence, the drive for transparency serves as a crucial strand in the fabric that weaves together machine learning practices.
Comprehending Predictive Outcomes in Artificial Intelligence
Understanding AI predictions is a critical aspect of unveiling the power and scope of Artificial Intelligence. These predictions, generated through analytical evaluations and intricate algorithms, often form the essence of AI's capacity to interpret, analyze, assess, and predict human behavior, market trends, consumer patterns, and much more. Thus, it becomes of paramount importance to comprehend these predictive outcomes in AI, not just from a technical perspective, but also from a business standpoint.
Within the ambit of Artificial Intelligence, predictive analysis is a dynamic arena that constantly evolves with changing trends and paradigms. This domain revolutionizes the way businesses operate, as data-driven insights can significantly impact decision-making processes, boosting organizational efficiency and driving innovation. Hence, understanding AI predictions is an integral part of the holistic approach towards deciphering the immense potential of AI in transforming conventional business models.
Promoting Accountability in AI Through Explainable Techniques
The advancement of Artificial Intelligence (AI) systems brings about complex decision-making processes that can often appear as a "black box" to their human users. This lack of transparency not only hinders trust in AI systems, but it also puts into question the issue of accountability when decisions made by these AI systems have real-world consequences. Enhancing AI accountability therefore becomes a crucial aspect, and this is where Explainable AI (XAI) techniques come into play.
Explainable AI elucidates the internal workings of AI systems in a way that is comprehensible to humans. By doing so, it enables users to understand and trust the predictions and decisions made by these systems. This transparency bridges the gap that often exists between AI developers and users, promoting accountability as it allows for the tracking of decision-making processes. Thus, through the implementation of XAI techniques, the ambition of enhancing AI accountability can be efficiently achieved, ensuring that AI systems are not only powerful but also respectful of ethical standards.
Leveraging Explainable AI for Advanced Predictive Analytics
Incorporating XAI, or Explainable Artificial Intelligence, in predictive analytics paves the path for innovative strategies. There is increased interest in the potential of XAI for predictive analytics, not only for its prowess in developing accurate predictions but also for offering comprehensive insight into the decision-making processes behind these predictions. This intersection of advanced analytics and transparency allows businesses to tap into their data in a way that is both efficient and ethically responsible.
Moreover, it is noteworthy that XAI's attribute of explainability can have far-reaching implications for predictive analytics. It fosters a sense of trust in the generated results, enabling stakeholders to validate and understand the intricacies of machine learning models. The power of XAI for predictive analytics lies in its ability to predict and detail simultaneously, providing a new approach to data-driven decision making. The state-of-the-art predictive models made by XAI add another dimension of transparency, reducing the opacity often seen in traditional AI, leading the organizations towards a trustworthy and secure data analysis journey.
Maintaining Ethical Standards in AI Predictions
The optimization of Ethical AI Predictions is crucial in the advancement of modern technology. As Artificial Intelligence (AI) permeates various facets of our daily lives, it is vital to ensure that the AI systems we deploy perform their tasks in a responsible, ethical manner. This means they should make predictions that respect societal norms, uphold human rights, and comply with existing regulations while also ensuring fairness, justice, and equitable outcomes for all users.
However, ensuring this ethical standard in AI predictions is not without its challenges. For one, the nature of AI itself often makes it difficult to define and interpret what an ethical outcome might look like. Furthermore, there is also the question of accountability: should an AI system make an erroneous or harmful prediction, determining who – or what – is to blame is a complex issue. Therefore, it is crucial to design AI models with a robust ethical framework upfront. This includes providing explicit guidelines for what constitutes ethical behavior, developing sophisticated processes for auditing and oversight, and establishing clear accountability mechanisms.
What is Explainable AI?
Explainable AI, often abbreviated as XAI, is a subset of artificial intelligence that emphasizes on making the decision-making process of AI models understandable and transparent to humans. This is crucial in maintaining ethical standards in AI predictions.
Why is transparency important in predictive models?
Transparency in predictive models is critical as it allows users to understand how the model functions, the logic behind its predictions, and the factors influencing outcomes. This enhances trust in the system and promotes accountability and ethical usage of AI.
How does Explainable AI contribute to establishing trust in Artificial Intelligence systems?
Explainable AI fosters trust in AI systems by providing clear, understandable explanations of how a decision or prediction was made. This transparency allows users to feel confident in the system's actions, knowing that it operates in an understandable and predictable manner.
Can you elaborate on the decision-making process in AI?
The decision-making process in AI involves the system analyzing inputs, running them through an algorithmic model, and producing a result or prediction. Explainable AI seeks to make this process transparent by detailing what factors influenced the final decision, making it easier for users to understand the AI's reasoning.
What are some key techniques in implementing Explainable AI?
Key techniques for implementing Explainable AI include feature importance, partial dependence plots, LIME, SHAP, and counterfactual explanations. These techniques help reveal the inner workings of the AI model, contributing to transparency and understanding.
Why is transparency pivotal in Machine Learning?
Transparency in machine learning is essential to ensure that the system's decisions can be understood, trusted, and, if necessary, contested. It helps prevent misuse, biases, and discrimination, which may arise due to opaque algorithms.
How can we comprehend predictive outcomes in Artificial Intelligence?
Through Explainable AI techniques, predictive outcomes in AI can be comprehended. These techniques present the reasoning behind the AI's decisions in an understandable manner, explaining what factors led to the prediction or output.
How does Explainable AI promote accountability in AI?
Explainable AI promotes accountability by making the decision-making process transparent. It enables users to understand the logic behind AI's decisions, facilitating checks and balances and ensuring that the system is being used ethically and responsibly.
How can Explainable AI enhance advanced predictive analytics?
Explainable AI can enhance advanced predictive analytics by providing clear insights into the decision-making process. This allows for better interpretation of results, validation of models, and ultimately, more informed decision-making.
How is maintaining ethical standards in AI predictions achievable?
Maintaining ethical standards in AI predictions is achievable through the implementation of Explainable AI. It provides transparency in the decision-making process, facilitates trust, promotes accountability, and ensures that the AI systems are used ethically and responsibly.
About the Author:
Waqar Jadoon, with 28 years in aviation supply chain management, holds an MBA and a software engineering degree. Renowned for streamlining operations and integrating AI and data analytics, he blends technical and business expertise to drive industry innovation. Waqar champions sustainable practices and technological advancements, shaping the future of aviation logistics
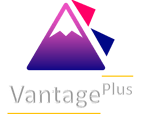

Location
Business Center 1, M Floor. The Meydan Hotel, Nad Al Sheba, Dubai U.A.E
Hours
Monday - Sunday 08:00am - 08:00pm
Contacts
Phone: +971566121732 info@vantageplusanalytics.com
waqarjadoon@vantageplusanalytics.com
FAQ